All evidence
Comparison of diagnostic performance of a deep learning algorithm, emergency physicians, junior radiologists and senior radiologists in the detection of appendicular fractures in children
Published in
Springer Nature
March 2023
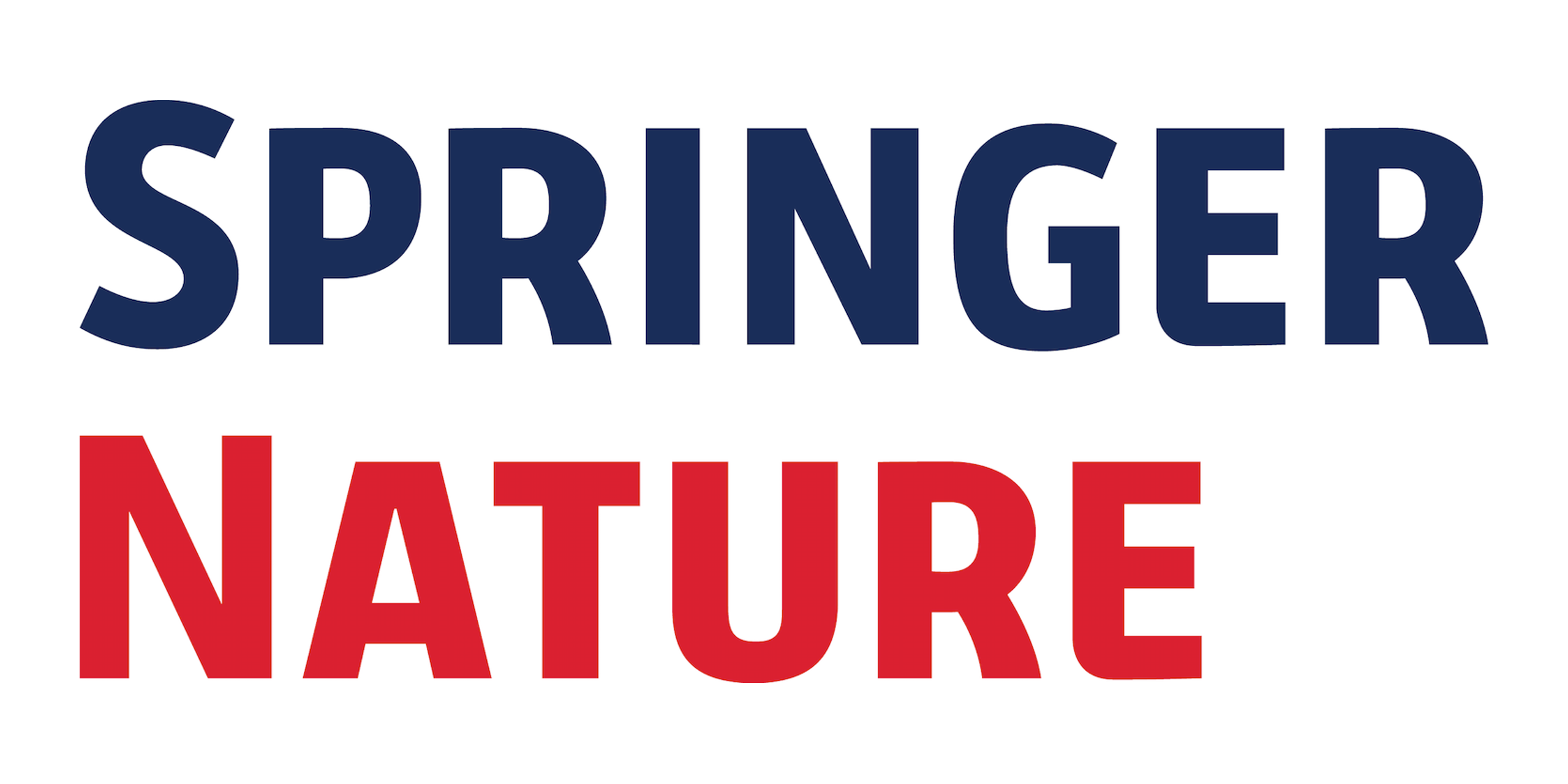
Authors
Idriss Gasmi, Arvin Calinghen, Jean-Jacques Parienti, Frederique Belloy, Audrey Fohlen, Jean-Pierre Pelage
Objective
To evaluate the performance of an AI algorithm based on deep neural networks toward detecting traumatic appendicular fractures in a pediatric population. To compare sensitivity, specificity, positive predictive value and negative predictive value of different readers and the AI algorithm.
Methods
This retrospective study conducted on 878 patients younger than 18 years of age evaluated conventional radiographs obtained after recent non-life-threatening trauma. All radiographs of the shoulder, arm, elbow, forearm, wrist, hand, leg, knee, ankle and foot were evaluated. The diagnostic performance of a consensus of radiology experts in pediatric imaging (reference standard) was compared with those of pediatric radiologists, emergency physicians, senior residents and junior residents. The predictions made by the AI algorithm and the annotations made by the different physicians were compared.
Results
The algorithm predicted 174 fractures out of 182, corresponding to a sensitivity of 95.6%, a specificity of 91.64% and a negative predictive value of 98.76%. The AI predictions were close to that of pediatric radiologists (sensitivity 98.35%) and that of senior residents (95.05%) and were above those of emergency physicians (81.87%) and junior residents (90.1%). The algorithm identified 3 (1.6%) fractures not initially seen by pediatric radiologists.
Conclusion
This study suggests that deep learning algorithms can be useful in improving the detection of fractures in children.
Our Rayvolve® AI Suite
CE
fda
trauma
CE
fda
83%
Turnaround Time reduction
67%
False Negatives reduction
99.7%
Negative predictive value
CE
chest
CE
36%
Reading Time reduction
11%
Sensitivity improvement
97.9%
Negative predictive value
CE
measures
CE
1.4°
Average MAE for Angles
1.3mm
Average MAE for Lengths
coming soon
bone age
COMING SOON
Based on Greulich & Pyle reference methodology
Statistical comparison with chronological age
Latest evidence
Optimize Your Workflow and Improve Quality of Care with AZmed
Discover the power of our AI Suite today!