All evidence
Assessing the Potential of a Deep Learning Tool to Improve Fracture Detection by Radiologists and Emergency Physicians on Extremity Radiographs
Published in
Academic Radiology
November 2023
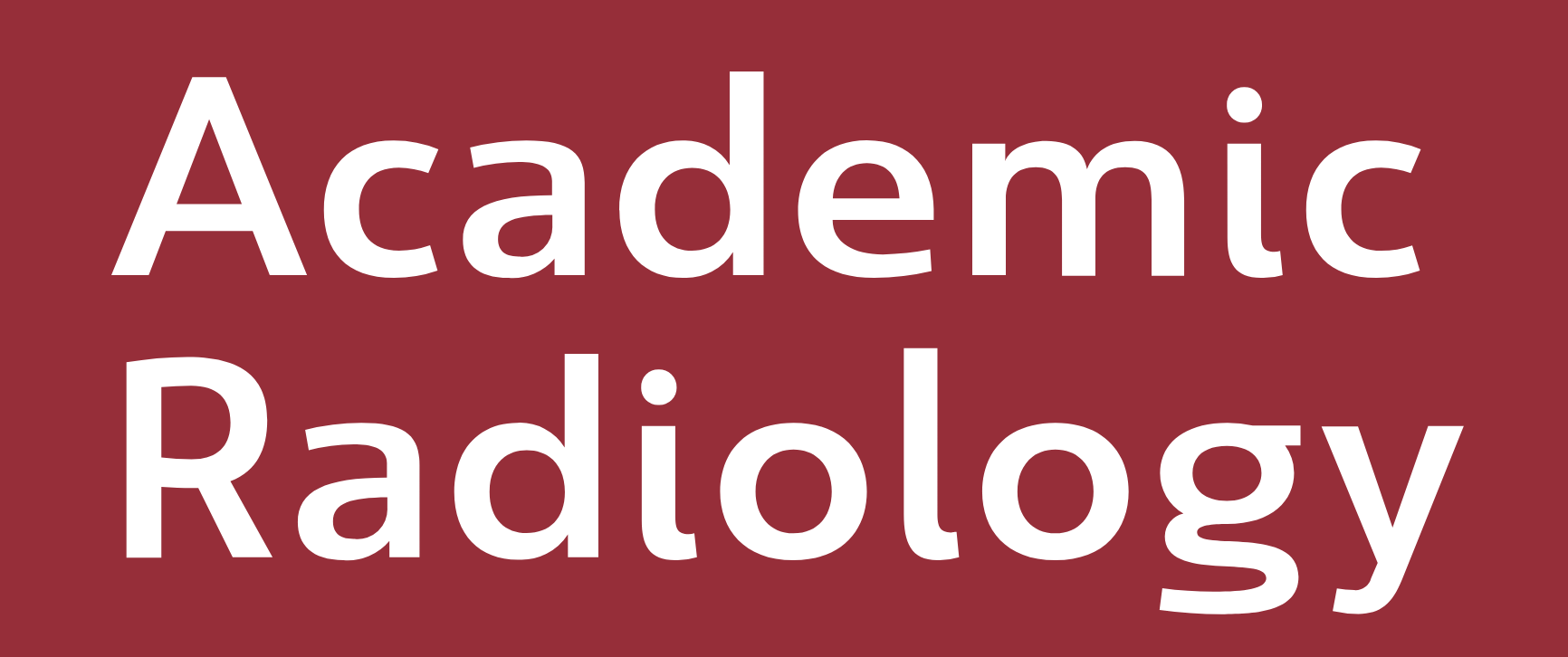
Authors
Tianyuan Fu, Vidya Viswanathan, Alexandre Attia, Elie Zerbib-Attal, Vijaya Kosaraju, Richard Barger, Julien Vidal, Leonardo K Bittencourt, Navid Faraji
Objective
To evaluate the standalone performance of a deep learning (DL) based fracture detection tool on extremity radiographs and assess the performance of radiologists and emergency physicians in identifying fractures of the extremities with and without the DL aid.
Methods
The DL tool was previously developed using 132,000 appendicular skeletal radiographs divided into 87% training, 11% validation, and 2% test sets. Stand-alone performance was evaluated on 2626 de-identified radiographs from a single institution in Ohio, including at least 140 exams per body region. Consensus from three US board-certified musculoskeletal (MSK) radiologists served as ground truth. A multi-reader retrospective study was performed in which 24 readers (eight each of emergency physicians, non-MSK radiologists, and MSK radiologists) identified fractures in 186 cases during two independent sessions with and without DL aid, separated by a one-month washout period. The accuracy (area under the receiver operating curve), sensitivity, specificity, and reading time were compared with and without model aid.
Results
The model achieved a stand-alone accuracy of 0.986, sensitivity of 0.987, and specificity of 0.885, and high accuracy (> 0.95) across stratification for body part, age, gender, radiographic views, and scanner type. With DL aid, reader accuracy increased by 0.047 (95% CI: 0.034, 0.061; p = 0.004) and sensitivity significantly improved from 0.865 (95% CI: 0.848, 0.881) to 0.955 (95% CI: 0.944, 0.964). Average reading time was shortened by 7.1 s (27%) per exam. When stratified by physician type, this improvement was greater for emergency physicians and non-MSK radiologists.
Conclusion
The DL tool demonstrated high stand-alone accuracy, aided physician diagnostic accuracy, and decreased interpretation time.
Our Rayvolve® AI Suite
CE
fda
trauma
CE
fda
83%
Turnaround Time reduction
67%
False Negatives reduction
99.7%
Negative predictive value
CE
chest
CE
36%
Reading Time reduction
11%
Sensitivity improvement
97.9%
Negative predictive value
CE
measures
CE
1.4°
Average MAE for Angles
1.3mm
Average MAE for Lengths
coming soon
bone age
COMING SOON
Based on Greulich & Pyle reference methodology
Statistical comparison with chronological age
Latest evidence
Optimize Your Workflow and Improve Quality of Care with AZmed
Discover the power of our AI Radiology Suite for X-rays today!